Methods
Atlas of Population Mobility in Czechia
(Atlas 2023
) is a collection of predicted data about population mobility in Czechia. Our primary objective in producing the Atlas 2023
is to preserve knowledge and reference values that will be useful for researchers, policymakers, and other stakeholders in the field of regional and transport geography. It contains information on the movement of people within the country delivered by two core datasets. Vertex Attribute Dataset
which contains information about the attributes or categories of each vertex in the graph, such as whether it is a commuter or a non-commuter. Edge Commute Dataset
which contains information about the commute relations between vertices in the graph, such as the number of people commuting between different pairs of vertices. See Atlas Course to understand the product development phases and Help Results for example BI data product content and definitions of specific terms.
Data Collection¶
The data collection process spanned nearly four years, preceded by a one-year testing period. The final time series covers the period from July 2019 to December 2022, ensuring a comprehensive dataset. Data from October 2019 to December 2022 are presented. The data processing methods remained consistent throughout the entire time span. The territorial scope of the data is the Czech Republic.
The dataset provides an expected representation of the entire population's mobility on average weekdays each month. The data has been predicted and calibrated to encompass the entire population of the Czech Republic, which consists of approximately 10.6 million inhabitants. There was a constant input in the model concerning the nominal population estimate values1. Outbound and inbound country travels are neglected. The spatial resolution of the data includes Regions
(14 in total) and District counties
(77 in total) within the Czech Republic. Additionally, for the vertex attribute dataset, data is available for Municipalities with population more than 38.000
, based on the CZSO2 classification. This includes 26 out of a total of 6,249 municipalities in the country.
The core data variables are supplemented with service variables such as GPS location and a standardized code list, facilitating easy implementation and integration with other data sources. Furthermore, where appropriate and available, the dataset includes reference values from official statistics as integral components.
Legal Status of Atlas 2023¶
The Atlas of population mobility in Czechia (Atlas 2023
) is not considered official statistics or a census conducted by a statistical office. It is important to note that Atlas 2023
is not an official publication of a governmental statistical agency. Instead, it is a separate project that involves the collection, processing, and analysis of data from various sources. While efforts are made to ensure the accuracy and reliability of the data, it does not have the same official status as data produced by a recognized statistical office or a national census. The data included in Atlas 2023
is designed to be compatible with the census data, meaning that it can be used in conjunction with the official statistics provided by the census. Atlas 2023
offers a different analysis of the data, showcasing the development of variables over time and exploring specific categories that provide additional understanding of the subject matter. These perspectives and categories of variables can help researchers, policymakers, and other stakeholders gain deeper insights into various phenomena or trends related to the data.
Czech Statistical Office Data Integration¶
We use variable called commutes_census
("dojizdka_celkova") to provide a comparative view of census statistics 3 to commutes
estimated by VSB-TUO. Due to the questions asked in the census, we only compare the one-way morning commutes. It's important for the reader to keep in mind that the census studied commutes to work and schools. The commutes presented in Atlas 2023
reflect the strongest destinations during the daily cycle and the return from these destinations. The difference between the two is apparent. Our model does not seek to identify the specific type of activity, nor does it distinguish the socio-economic purpose of the commutes. Another notable difference is the frequency. We extrapolate and predict data based on an average weekday in a month. The census asks a yes-or-no type of question about commuting to work and school, and an additional question focuses on the frequency of commutes, which is evaluated as a separate statistic 4.
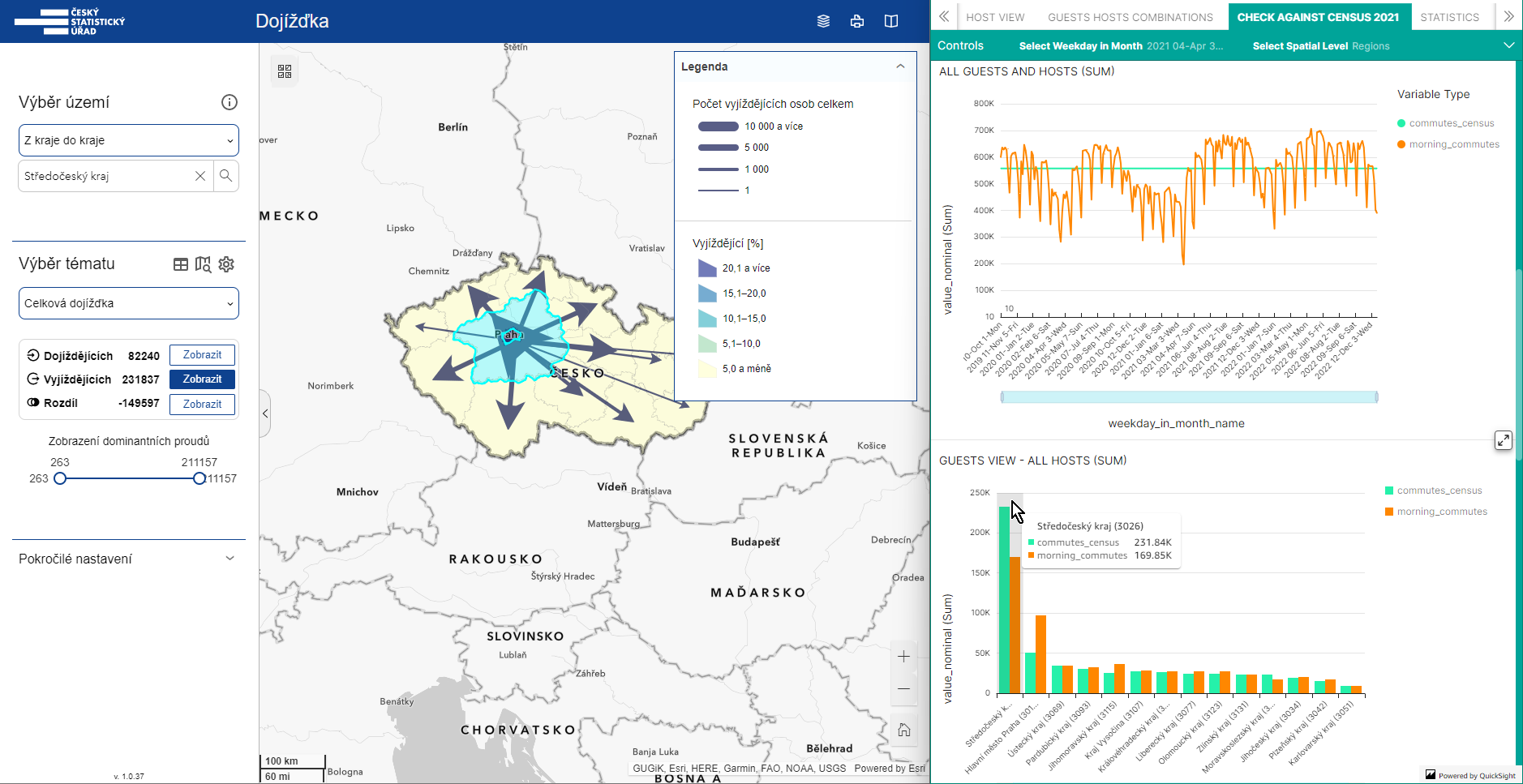
Left Aplikace Dojížďka (CZSO), right SESSION EDGE COMMUTE WEEKDAYS (VSB-TUO) with settings to year 2021, month April, weekdays Wednesdays.
Data Accessibility¶
The data included in Atlas 2023
is accessible to the general public. Basic Business Intelligence (BI)
functionality is enabled, allowing users to interact with the data and explore predefined visualizations and reports. Additionally, the dataset can be downloaded for customer proprietary analyses. This means that users have the option to access and utilize the data for their own specific analyses and investigations. The dataset can be obtained through the AWS Marketplace, providing a platform for users to acquire and work with the data according to their needs. By making the data accessible through these means, Atlas 2023
aims to promote transparency and facilitate further exploration and analysis by researchers, analysts, and individuals interested in the subject matter.
Difference of Data Published in Atlas 2023 to Data Delivered Within Customer´s Projects¶
The scope of the published data in Atlas 2023
differs from the data delivered within specific customer projects. While the customer projects may have focused on specific requirements or areas of interest, the Atlas 2023
aims to provide a holistic view on the territory of the Czech Republic, using interpolated and predicted data for selected variables. It incorporates insights, experiences and findings from various sources and studies to present a comprehensive understanding of the subject matter. Data published in Atlas 2023
can be seen as a source that offers a different perspective compared to the data delivered within individual customer projects.
Methods of Calculations for Finished Customer´s Projects¶
To ensure effective archiving, we present an excerpt of the calculation methods employed in the projects that contributed to the findings, experiences and understandings presented in Atlas 2023
.
Population mobility pattern
refers to the patterns of movement of people within a certain area or region. This can include information on migration (movement between different regions or countries), commuting (movement between home and work), and internal migration (movement within a country or region). It also can refer to the characteristics of the people who are moving, such as their age, gender, education level, and economic status. More specifically Population mobility commuting patterns in daily cycle
refers to the patterns of movement of people during the course of a day, specifically related to commuting to work, school or home (commuting origins). It describes the time of day, direction and the mode of transportation used for commuting by people. This information helps to understand how people travel to and from these stations, and how this changes over the course of the day. In our interpretation one group may consist of people who commute to work or school in the morning (Morning Commutes
), while another group may consist of people who commute home in the evening (Evening Commutes
).
The Daily cycle
for Classification of population by mobility commuting patterns in daily cycle
has 24 hours and is the basis for the classification of SIM users from the point of view of mobility. This is the cycle of being awake during the day and sleeping at night that is normal for most of the population. In this context Stations of Significant Occurrence in Daily Cycle
is defined for morning, day and evening. For analytical purposes we define time windows for morning as 00:00:00 - 04:59:59, day as 05:00:00 - 08:59:59 and evening as 19:00:00 - 23:59:59. The Classification of population by mobility commuting patterns in daily cycle
is based on a retrospective evaluation of SIM movement information in the mobile network. The movement of the SIM is monitored by means of signaling messages for the establishment, maintenance and termination of the mobile connection, which are exchanged between the SIM located in the telecommunications device, typically a mobile phone, and transmitters deployed in the territory. The classification is made exclusively from the point of view of the mobility detected in this way, i.e. the fact of occurrence in the territory detectable with a given probability from the signaling of the mobile network. No additional data and assumptions are used for the classification.
Classification of population by mobility commuting patterns in daily cycle
-
By
Classification of population by mobility commuting patterns in daily cycle
we understand simplified classification by patterns of movement between home and school or work, school or work and home, shool or work and other place then home. For home we understandStation of Home Stay in Daily Cycle
, for school or work we understandStation of Commutes in Daily Cycle
and for other place then home we understandStation of Significant Occurrence in Evening Time Window
. We emphasize simplified in a sense that by no means we attempt to determine if the station is for given person home, school, etc. We simply follow the patter first station - second station - first station or third station and get so calledStations of Significant Occurrence in Daily Cycle
. -
We use following method for obtaining
Stations of Significant Occurrence in Daily Cycle
.Stations of Significant Occurrence in Daily Cycle
, represented by cell of the mobile network, is assigned to the user based on the total time, i.e. the total time the user spends in the cell of the mobile network during theDaily cycle
. The total time is calculated cumulatively for theDaily Cycle
, regardless of whether the user remained in the Mobile Network Cell continuously or changed the Mobile Network Cell during the day. In cases where the user changed the Cell of the mobile network, i.e. the SIM record was recorded on another Cell of the mobile network, the accumulation of time for the original Cell of the mobile network stops until the user logs in to this Cell of the mobile network again. By the method we get for each SIMStation of Significant Occurrence in Morning Time Window
,Station of Commutes in Daily Cycle
andStation of Significant Occurrence in Evening Time Window
. In caseStation of Significant Occurrence in Morning Time Window
andStation of Significant Occurrence in Evening Time Window
are similar, we call these stations byStation of Home Stay in Daily Cycle
(see detail method bellow) and SIMs which fulfilled this condition are classified asHusbandmen
. In caseStation of Significant Occurrence in Morning Time Window
andStation of Significant Occurrence in Evening Time Window
do not equal, but exist, SIMs which fulfilled this condition are classified asNomads
. -
Station of Home Stay in Daily Cycle
. The morning and evening windows are used to determine theStation of Home Stay in Daily Cycle
. First, all transmitters (BTS (2g), NodeB(3g), eNodeB(4g)) where the given user had at least one record are determined. At least one record of the same transmitter must occur in both the morning and evening windows. As the home transmitter, the one that, according to the records, spent the longest time in the evening and morning window is selected. Subsequently, the cell belonging to this transmitter is selected, where the user spent the longest time out of all other cells of this transmitter. This cell no longer needs to appear in both windows, the condition of the longest spent time is enough. The cell selected in this way is the input of theMathematical model of the conversion of observations in the mobile network to a territorial element or a specific territory
for calculatingStation of Home Stay in Daily Cycle
. -
Mathematical model of the conversion of observations in the mobile network to a territorial element or a specific territory
. The model enables the conversion of totals of signaling data detected through the mobile network to totals of SIM in the administrative division of the territory. SW works in two variants, namely (a) for a specific territory defined by any square polygon with a minimum size of 100 x 100 and (b) for an administratively divided territory defined by the spatial element of the basic residential unit dial or the spatial element of the smart territory dial with subsequent composition into higher territorial units. The main function of the model consists in connecting the polygons of the coverage model with the polygons of an arbitrarily defined territory and their territorial elements, taking into account the continuous updating of input parameters both on the side of the mobile network and on the side of territorial elements, and last but not least, their attributes determined by statistical methods. The model works with the coverage of the following networks: FDD is UMTS; 2100 MHz; GSM is 2G; 900 and 1800 MHz; l18 is 4G LTE; 1800MHz; l21 is 4G LTE; 2100 MHz; l26 is 4G LTE; 2600 MHz; l80 is 4G LTE; 800 MHz. There may be cells in the network that are represented by a very small polygon or their coverage polygon does not contain a building object. Records from these cells are neglected when applying the model to a land feature, unless otherwise specified. View the full model description in Czech language.
-
Počet obyvatel v obcích - k 1.1.2018. 3 2019. Accessed on June 30, 2023. URL: https://www.czso.cz/csu/czso/pocet-obyvatel-v-obcich-see2a5tx8j. ↩
-
Obyvatelstvo čsú. 2 2023. Accessed on February 28, 2023. URL: https://www.czso.cz/csu/czso/obyvatelstvo_lide. ↩
-
Dojížďka mezi obcemi, včetně denní dojížďky. 03 2021. Accessed on May 18, 2023. Dojížďka mezi obcemi, včetně denní dojížďky (data a schéma) 04.05.2023 (kód: 170359-23). URL: https://www.czso.cz/csu/czso/vysledky-scitani-2021-otevrena-data. ↩
-
Vyjíždějící do zaměstnání a školy podle frekvence vyjížďky. 03 2021. Accessed on May 18, 2023. URL: https://www.czso.cz/csu/czso/vysledky-scitani-2021-otevrena-data. ↩